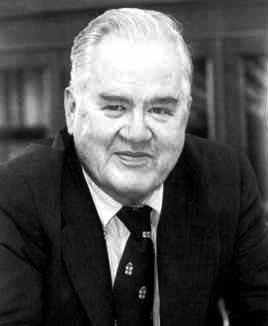
EDA: How Data Analysis Becomes a Necessary First Step for Big Data and Predictive Analytics Modeling
When a multidisciplinary research study group at Princeton University undertook a study of the paired uses of electricity and gas in townhouses, it contacted the residents of Twin Rivers, a nearby planned community in New Jersey. Over a five-year study period, it learned how to eliminate three-quarters of the energy used by the furnace in quite ordinary, reasonably well-built townhouses, as chronicled in Saving Energy in the Home: Princeton’s Experiments at Twin Rivers, edited by Robert H. Socolow (Cambridge, MA: Ballinger, 1977).
The purpose of the Princeton study, during a winter in the mid-1970s, was to examine differences in energy use and make comparisons with structural aspects of the 152 individual townhouses and the behavioral aspects of their inhabitants. As a data scientist (a.k.a. applied statistician), I took great delight in being a participant and was intrigued by later looking at the results and the data from the study. I was a resident at Twin Rivers at the time, not realizing that some new analysis techniques used on the data would eventually be published in 1977 in the ground-breaking book Exploratory Data Analysis by data science pioneer John W. Tukey (1915–2000).
The data were gathered automatically through a special device that was hooked up to the landline telephones and the energy sources in the home. There were questions to be answered periodically about our lifestyle, the details of which have long escaped my memory. Nevertheless, some novel uses of graphing techniques with schematic data plots (data visualization) can be found throughout this book. These techniques, new at the time, have now become a familiar part of many business statistics books.
Exploring Data Patterns
Studying the patterns in the data improves the forecaster’s chances of successfully modeling data for forecasting applications. Through exploratory data analysis (EDA), a demand forecaster can start the important task of finding factors (drivers of demand) that are generally quantitative in nature.
John Tukey likens EDA to detective work: “A detective investigating a crime needs both tools and understanding. If he/she has no fingerprint powder, the detective will fail to find fingerprints on most surfaces. If detectives do not understand where criminals are likely to have put their fingers, they will not look in the right places.” A planned forecasting and modeling effort that does not include provisions for exploratory data analysis often misses the most interesting and important results; but it is only a first step, not the whole story.
Exploratory data analysis means looking at data, absorbing what the data are suggesting, and using various summaries and display methods to gain insight into the process generating the data.
Demand planning is mostly about data. One of the key points that Hans Levenbach makes in this outstanding treatment of demand forecasting. I know that I have often been in a hurry to “start forecasting” without taking the time to really dissect the data available to help refine the forecasts. Lesson learned. It may be boring to spend time gathering and analyzing data; but the payoff in better understanding how to turn the numbers into conversations is vital. Daniel Fitzpatrick, Demand Planner
Chance&Chance Embraced, a 5-star book is available in paperback and e-book at Amazon.
Hans Levenbach, PhD is Executive Director, CPDF Training and Certification Professional Development Programs. He conducts hands-on Workshops on Smarter Forecasting for buness planners and managers in supply chain organizations worldwide. He is group manager of the LinkedIn groups (1) Demand Forecaster Training and Certification, Blended Learning, Predictive Visualization, and (2) New Product Forecasting and Innovation Planning, Cognitive Modeling, Predictive Visualization.
I invite you to join and comment if you have an interest in sharing conversations on these topics.