A New Way to Monitor Accuracy of Intermittent Lead-time Demand Forecasts: The Forecast Profile Performance (FPP) Index
Intermittency in demand forecasting is a well-known and challenging problem for sales, inventory and operations planners, especially in today’s global supply chain environment. Intermittent demand for a product or service appears sporadically with lots of zero values, and outliers in the demand data. As a result, accuracy of forecasts suffers, and how to measure and monitor performance has become more critical for business planners and managers to understand. The conventional MAPE (Mean Absolute Percentage Error) measure is inadequate and inappropriate to use with intermittency because of divisions by zero in the formula lead to undefined quantities.
In a previous article, I introduced a new approach, which can be more useful and practical than a MAPE in dealing with inventory lead-times , financial budget planning, and sales & operations (S&OP) forecasting. What these applications have in common are the multi-step ahead forecasts, whose accuracy needs to be assessed on a periodic basis. I will call this new approach the Forecast Profile Performance (FPP) process.
A Smarter Way to Deal with Intermittency in Demand Forecasting
For the past four to five decades, the conventional approach in dealing with intermittency has been based on the assumption that interdemand intervals and the nonzero demand events are independent. This is the logic behind the Croston method and its various modifications. But, when you start looking at real data in an application, you discover that this may not be valid.
This new approach examines the data in two stages. First, we observe that each demand event is preceded by an interdemand interval or duration. Thus, given a duration, we can assume that a demand event follows. In a regular demand history, each demand event is preceded by an interval of zero duration. Under this assumption, non-zero Intermittent Demand events ID* are dependent on ‘Lagtime’ Zero Interval durations LZI. The LZI distribution for our spreadsheet example is shown in the left frame. In the right frame, we show the conditional distribution for the ID* given the three LZI durations. They are not the same hence it would not be advisable to assume that they are.
When forecasting ID* for an inventory safety stock setting, for example, it will be necessary to consider each of the conditional distributions separately. In the spreadsheet above, I have created three forecasts for the 2017 holdout sample. Forecast F1 is a level naïve forecast with 304 units per month. For ease of comparisons, I have assumed that annual totals are the same as the holdout sample. In practice, this would not necessarily be true. The same is assumed for Forecasts F2 and F3. so that comparisons of forecast profiles are the primary focus. In practice, the effect of a ‘bias correction’, if multi-step forecast totals differ, should also be considered, However, consideration of the independence assumption is a fundamental difference between a Croston method and this one.
Step 1. Creating Duration Forecasts
When creating forecasts, we first need to forecast the durations. This should be done by sampling the empirical or assumed LZI distribution. In our spreadsheet, LZI_0 intervals occur more frequently than the other two and the LZI_1 and LZI_2 intervals occur with about the same frequency. Then, based on the LZI in the forecast, a forecast of ID* should be made from the data the LZI it depends on. An example of this process is given in an earlier post and on the Delphus website.
After a forecasting cycle, is completed and the actuals are known, both the LZI distribution and the conditional ID* distribution need to be updated before the next forecasting cycle, so the distributions can be kept current.
Step 2. Creating the Alphabet Distributions
Depending on your choice of a Structured Inference Base (SIB) model for intermittent demand ID* (or the natural log transformed intermittent demand Ln ID*), alphabet profiles need to be coded for the actuals and the forecasts from the (conditional) demand event distributions for each of the durations LZI_0 in column C, LZI_1 in column F, and LZI_2 in column I. Note that the sum of the weights in an alphabet profile add up to one.
For the data spreadsheet we need also the add the alphabet weights associate with the duration distribution. This is done by multiplying the conditional alphabet weights by the weights in the (marginal) duration distribution LZI_0 (= 0.4545), LZI_1 (= 0.2727), and LZI_2 (= 0.2727). These coded alphabet weights can be found in columns D, G, and J, respectively. Note that the sum of the three weights in the combined alphabet profiles add up to 1.
Step 3. Measuring the Performance of Intermittent Demand Profiles
In a previous article on LinkedIn as well as my website blogs, I introduced a measure of performance for intermittent demand forecasting that does not have the undefined terms problem arising from intermittency. It is based on the Kullback-Leibler divergence or dissimilarity measure from Information Theory applications.
where a(i ) (i = 1,2, …, m) are the components of the Actuals Alphabet Profile (AAP) and f(i) (i = 1,2, …, m) are the components of the Forecast Alphabet Profile (FAP), shown in the spreadsheet below. In the forecast, the lead-time, budget cycle, or demand plan is the horizon m.
Step 4. Creating a Forecast Profile Performance (FPP) Index
The alphabet profile for the actuals (AAP) is shown in the top line of the spreadsheet above. The alphabet profiles for the forecasts (FAP) are shown in the second, fourth, and sixth lines in the spreadsheet. The calculations for the D(a|f) divergence measure are shown in the third, fifth, and seventh lines with the D(a|f) results shown in bold. They are 22%, 40%, and 16%. respectively, for forecasts F1, F2, and F3. It can be shown that D(a, f) is non-negative and equal to zero, if and only if, the FAP = AAP, for every element in the alphabet profile. Hence, F3 is the most accurate profile followed by F1 and F2. This should not be interpreted to mean that F3 has the best model. However, it does suggest that the F3 model has the best multi-step ahead forecast pattern of the actuals. One should monitor D(a, f) for multiple forecasting cycles, as trend-seasonal patterns can change for monthly data, in this instance.
FPP (f) index = 100 + D(a|f)
We can create a Forecast Profile Performance index by adding 100 to D(a f), so that 100 is the base for the most accurate possible profile.
Step 5. Monitoring a FPP index.
The FPP index needs to be charted for every forecasting cycle, much like a quality control chart in manufacturing. One way of creating bounds for the index is to depict three horizontal bars (green, amber and red), where the lowest bar, starting at 100, is the limit for an acceptable accurate profile, the middle bar for profiles to be reviewed, and the red bar for unacceptable performance. The criteria for the bounds should all be based on downstream factors developed from your particular environment and application.
I invite you to share your thoughts and practical experiences with intermittent data and smarter demand forecasting in the supply chain. Feel free to send me the details of your findings, including the underlying data used without identifying descriptions, so I can continue to test and validate the process for intermittent data.
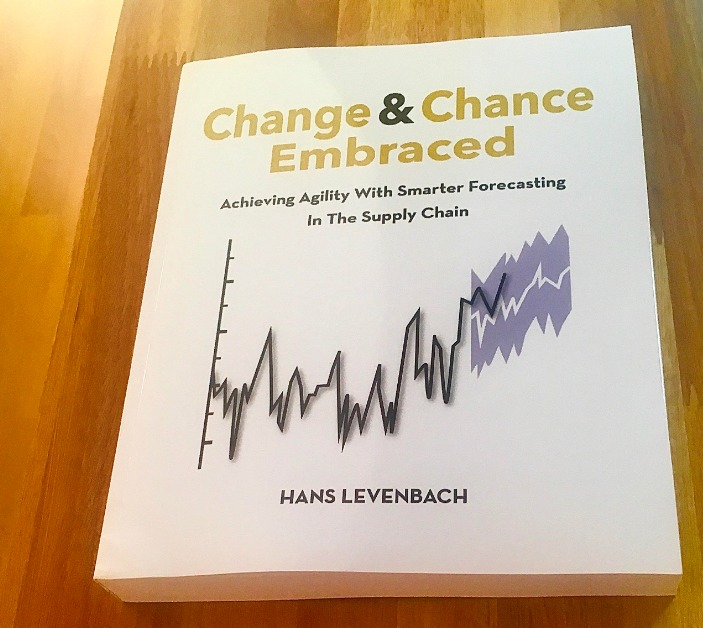